Slides presented at NCAF 2010 20 June
GP evolves parallel graphics card kernel for gzip
W. B. Langdon
CREST lab,
Department of Computer Science
Introduction
- Genetic Programming (GP).
- Using GP to create C source code
- Proof of concept: gzip on graphics card
- Template based on nVidia kernel
- BNF grammar
- Fitness
- Lessons (it can be done!)
Genetic Programming
- A population of randomly created programs
- whose fitness is determined by running them
- Better programs are selected to be parents
- New generation of programs are created by randomly combining above average parents or by mutation.
- Repeat generations until solution found.
Tree (A-10)*B
GP Generational Cycle
Creating new programs - Crossover
Some applications of Genetic Programming
- Most GP generates solutions, e.g.:
- Data modelling, soft sensors, design (circuits, lenses, radio aerial), image processing, predicting steel hardness, cinema: boids
- Recent use of GP for bug fixing.
- C code, zune bug, etc.
- The IFIP TC2 Manfred Paul Award for Excellence in Software: Theory and Practice
GP to write source code
- Software is labour intensive
- When to use GP to create source code
- Small. E.g. glue between systems.
- Hard problems. Many skills needed.
- Multiple conflicting ill specified non-functional requirements
- GP as tool. GP tries many possible options. Leave software designer to choose between best.
GP Automatic Bug Fixing
- Able to localise where bug might be.
- Need a test case to demonstrate bug
- Small number of tests prove fixed code still works.
- Replace C source with randomly chosen human written code.
- Test candidate bug fixes. Select better. Create new population. Loop.
- Clean up/validate bug fix.
GP Automatic Coding
- Target small unit.
- Use existing system as environment holding evolving code.
- Use existing test suite to exercise existing system but record data crossing interface.
- Use inputs & answer (Oracle) to train GP.
- How to guide GP initially?
- Clean up/validate new code
GP Automatic Coding
- Actual data into and out of module act as de facto specification.
- Evolved code tested to ensure it responds like original code to inputs.
- Recorded data flows becomes test Oracle.
Proof of Concept: gzip
- Example: compute intensive part of gzip
- Recode as parallel CUDA kernel
- Use nVidia’s examples as starting point.
- BNF grammar keeps GP code legal, compliable, executable and terminates.
- Use training data gathered from original gzip to test evolved kernels.
- Why gzip
- Well known. Open source (C code). SIR test suite. Critical component isolated. Reversible.
CUDA 2.3 Template
- nVidia supplied 67 working examples.
- Choose simplest, that does a data scan. (We know gzip scans data).
- Naive template too simple to give speed up, but shows plausibility of approach.
- NB template knows nothing of gzip functionality. Search guided only by fitness function.
scan_naive_kernel.cu
WBL 30 Dec 2009 Revision: 1.11 Remove comments, blank lines. int g_odata, uch g_idata. Add strstart1 strstart2, const.
move offset and n, rename n as num_elements
WBL 14 r1.11 Remove crosstalk between threads threadIdx.x, temp -> g_idata[strstart1/strstart2]
__device__ void scan_naive(int *g_odata, const uch *g_idata, const int strstart1,
const int strstart2)
{
int thid = 0; //threadIdx.x;
int pout = 0;
int pin = 1;
int offset = 0;
int num_elements = 258;
<3var> = (thid > 0) ? g_idata[thid-1] : 0;
for (offset = 1; offset < num_elements; offset *= 2)
{
pout = 1 - pout;
pin = 1 - pout;
<3var> = g_idata[strstart+pin*num_elements+thid];
if (thid >= offset)
<3var> += g_idata[strstart+pin*num_elements+thid - offset];
}
return <3var> ;
}
BNF grammar
scan_naive_kernel.cu converted into grammar (169 rules) which generalises code.
<line10-18> ::= "" |<line10-18a>
<line10-18a> ::= <line10e> <line11> <forbody> <line18>
<line11> ::= "{\n" "if(!ok()) break;\n"
<line18> ::= "}\n"
<line10e> ::= <line10> |<line10e1>
<line10e1> ::= "for (offset =" <line10.1> ";" <line10e.2> ";offset" <line10.4> ")\n"
<line10.1> ::= <line10.1.1> |<intexpr>
<line10.1.1> ::= "1" |<intconst>
<line10e.2> ::= <line10e.2.1> |<forcompexpr>
<line10e.2.1> ::= "offset" <line10.2> <line10.3>
<line10.2> ::= "<" |<compare>
<line10.3> ::= <line10.3.1> |<intexpr>
<line10.3.1> ::= "num_elements" |<intconst>
<line10.4> ::= "*= 2" |<intmod>
<intmod> ::= "++" |<intmod2>
<intmod2> ::= "*=" <intconst>
Fragment of 4 page grammar
gzip
- gzip scans input file looking for strings that occur more than once. Repeated sequences of bytes are replaced by short codes.
- n2 reduced by hashing etc. but gzip still does 42 million searches (sequentially).
- Demo: convert CPU hungry code to parallel GPU graphics card kernel code.
gzip longest_match()
Fitness
- Instrument gzip.
- Run gzip on SIR test suite. Log all inputs to longest_match(). 1,599,028 records.
- Select 29,315 for training GP.
- Each generation uses 100 of these.
Number of Strings to Check
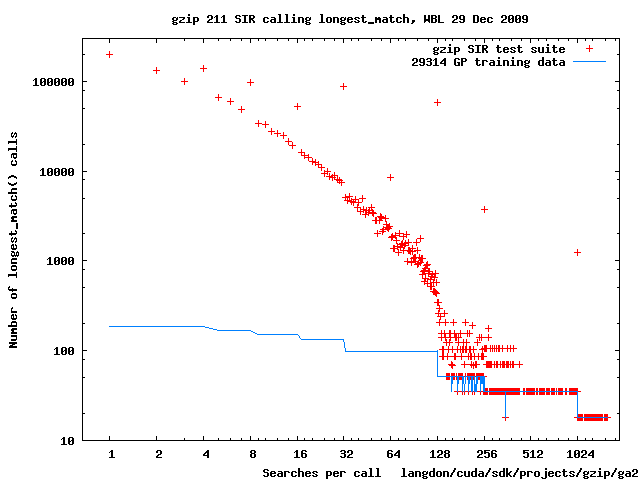
gzip hash means mostly longest_match() has few strings to check.
Training data more evenly spread.
Length of Strings to Check
1% 0 bytes
0% 1 bytes
0 2 bytes
30% 3 bytes
26% 4 bytes
25% 5 bytes
14% 6 bytes
gzip heuristics limit search ≤ 258
Fitness 2
- The tests are run on the original gzip code and its answers saved.
- Each evolved CUDA kernel (1000) is run and answers compared with gzip's answer. Up to 1588000 threads.
- performance = Σ|error| + penalty
- Many kernels always return 0, these get high penalty.
Performance of Evolved Code
Fall in number of poor programs
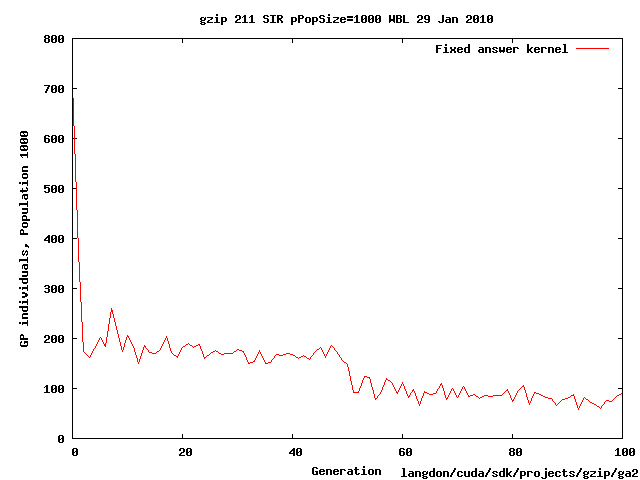
71% useless constants in generation 0
7% constants in last generation
nVidia GeForce 9600 GT
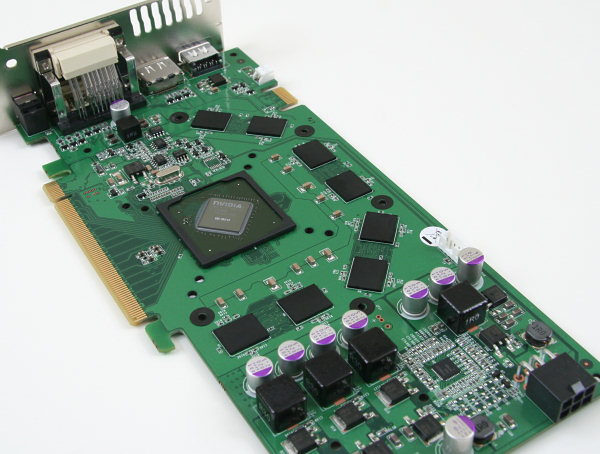
64 Stream Processors
Clock 1.5 GHz
512 MByte
7.8×4⅜ inches
Available
4 Tesla up to 16GBytes
Fermi 64 bit
512 processors
3 billion transistors
Laptops too
108 CUDA computers
Evolution of program complexity
Evolution of gzip Kernel
Strongly typed grammar based GP behaving like conventional tree GP
Evolved gzip matches kernel
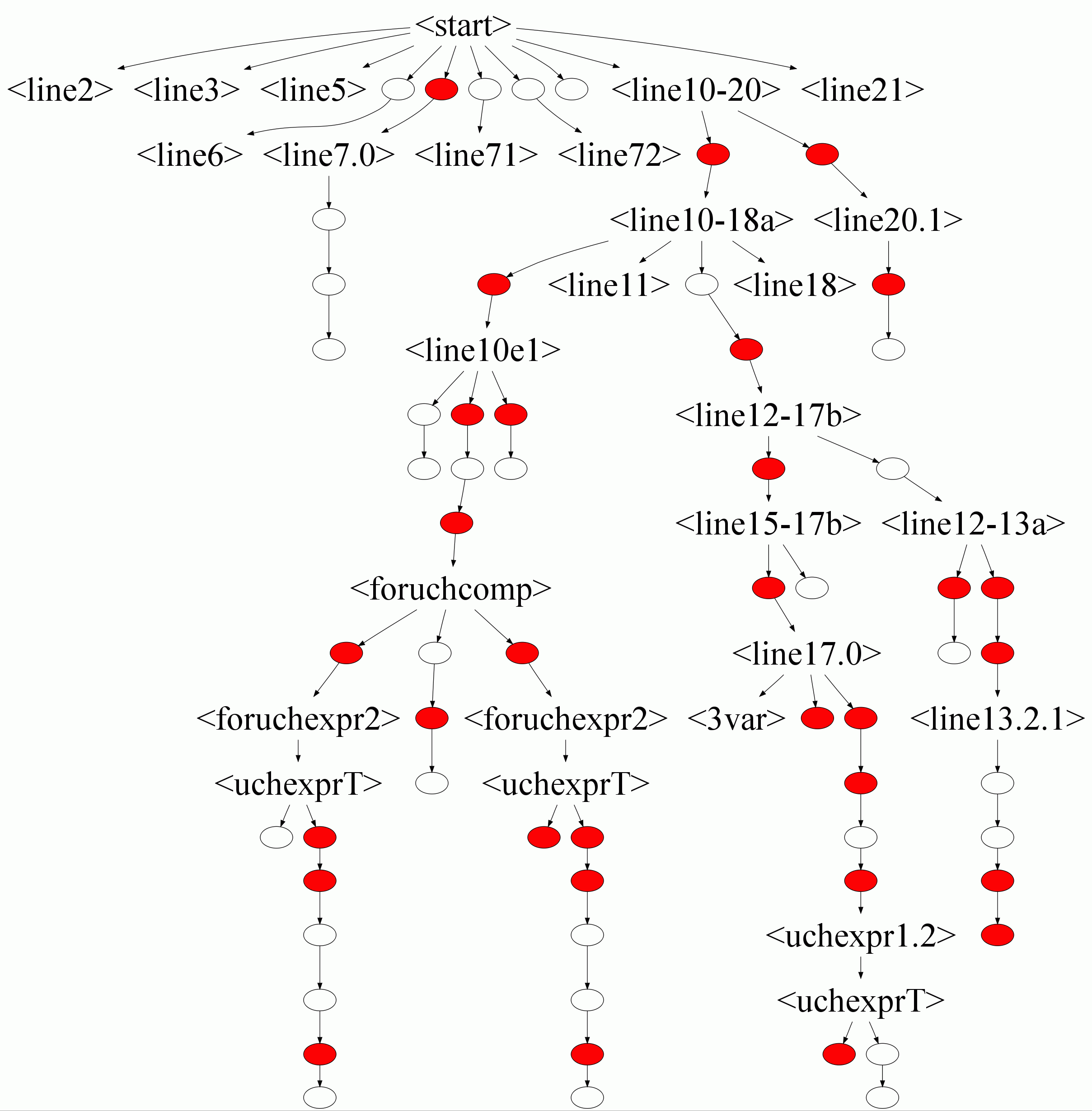
Parse tree of solution evolved in gen 55.
Ovals are binary decision rules. Red 2nd alternative used.
Evolved gzip matches kernel
__device__ int kernel978(const uch *g_idata, const int strstart1, const int strstart2)
{
int thid = 0;
int pout = 0;
int pin = 0;
int offset = 0;
int num_elements = 258;
for (offset = 1 ; G_idata( strstart1+ pin ) == G_idata( strstart2+ pin ) ;offset ++ )
{
if(!ok()) break;
thid = G_idata( strstart2+ thid ) ;
pin = offset ;
}
return pin;
}
Blue -fixed by template.
Black -default
Red -evolved
Grey - evolved but no impact.
Conclusions
- Have shown possibility of using GP to automatically re-engineer source code
- Problems:
- Will users accept code without formal guarantees?
- Evolved code passes millions of tests.
- How many tests are enough?
- First time code has been automatically ported to parallel CUDA kernel by an AI technique.
Langdon+Harman
WCCI 2010